The 3 reasons why AI projects tend to fail, and how to overcome them
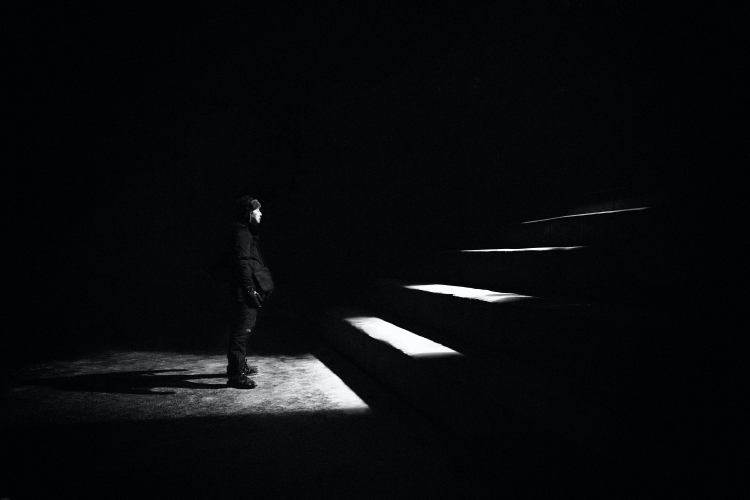
According to the Paetra whitepaper from 2019, nearly 85% of Artificial Intelligence (AI) projects fail and around 75% of the respondents say that early AI projects underwhelm.
Gartner also concurs with a similar prediction: 85% of AI projects won’t deliver for their sponsors.
Reading these surveys, it is no wonder that the startup scene is littered with failed AI companies that did not connect with the market. While some look good on paper when it comes to the actual adoption, these experimental systems fall short of delivering meaningful results in the real world and get discarded for it.
Unfortunately, the consensus seems to be that projects with AI components are more likely to fail than succeed. But why is that?
In my experience, there are a few fundamental reasons why AI projects fail:
- First, The ever-expanding field of AI
- Secondly, Merging Natural and Artificial Intelligence
- And finally; “We’ll figure it out as we go" mentality
By the end of this read, you’ll have a few takeaways on how to mitigate them.
The ever-expanding field of AI
The field of AI has experienced numerous advancements during recent decades, exhibiting promising results in many industries. One good example of the widespread of AI is the number of AI/ML related publications. For example, in Aalto university, the yearly research output has octupled since 2010.
Because AI is used in so many ways and by so many people with various backgrounds, the term has escalated to cover almost anything from statistics to hand-coded if–then expressions, depending on who you ask. Moreover, it is constantly evolving term that is redefined.
Solutions that might have been called AI only 10 years ago, are now so conventional, and thoroughly researched, that they are not even considered AI – rather new fundamentals of modern computer science.
John McCarthy, who coined the term “Artificial Intelligence” in 1956, famously said that “as soon as it works, no one calls it AI anymore”, and evidently this still rings true.
As the field of AI has expanded rapidly, keeping up with the ever-developing tools and technologies has become more challenging. The more expensive and complicated the tool, the more important it is to learn how to use it correctly – as successful implementation of AI requires a deep understanding of different technologies, their possibilities and limitations.
Merging Natural and Artificial Intelligence
Adding the word “intelligence” applies an additional level of “humane" complexity to software.
“(Intelligence) -...can be described as the ability to perceive or infer information, and to retain it as knowledge to be applied towards adaptive behaviors within an environment or context.” – Wikipedia, Intelligence
Intelligence in computers or other machines is called artificial. Unlike the naturalintelligence displayed by humans and animals, which involves consciousness and emotionality.
The difficulty in interpretation arises when the word intelligence is used without explicitly partitioning it from either category. One might say that software is intelligent, perhaps because an AI component within it recognizes forged signatures. But by saying so would suggest that the carefully optimized process might be capable of performing any task an intelligent person is able to perform, enforcing potential misunderstandings.
Working towards impossible goals or “lack of imagination” with identifying new use cases, are the symptoms of perceiving the two (Natural and Artificial Intelligence) as a singular “Intelligence”. When you understand for what a hammer is used for, you’ll not be using it to tighten screws.
"We'll figure it out as we go" mentality
Organizations are faced with problems that are completely opposite to the ones they confronted a few years ago. Rather than a small number of complex and expensive AI applications, they can now choose from a wide range of adaptable, easy-to-implement and affordable ones.
Cloud services can be quickly integrated into existing workflows; automating specific stages of a business process provides an easy and incremental path for organizations to evolve e.g., from simple task automation to intelligent automation, if applied correctly.
However, with so many options most jump on the AI bandwagon by picking a technology with much optimism, without a clear strategy and consideration on the most fundamental risks associated with AI.
Delivering AI is not just about programming the solution. It requires a strategic approach, with clear objectives and KPI/ROI tracking. Otherwise, you won’t be able to assess the results delivered by AI and compare them with your assumptions in order to measure the success (or failure) of this investment.
Takeaways
- If you do not have plans to evaluate AI, it’s time to think about catching up and educating your organization. Institutional understanding remains the biggest barrier in AI adoption. If you think AI can help, you should spend time explaining how, why, and what to expect.
- A common challenge seems to be in determining the right AI solution and aligning implementation with clearly defined business goals. So, take small steps first when assessing AI cases. Open dialogue with expert service providers can be an educating first step. This way, you’ll see whether they understand your business, have the right skills, and know-how to address your pain points.
Concluding thoughts
According to our experience, many executives perceive AI as a both business driver and a strategic differentiator and it should be a key investment in organizations’s future toolboxes. However, it’s easy to get lost in the variety of solutions and used terms.
It’s important to remember that Implementing AI is not just about choosing the right tool, programming the solution or buying the service. The right move is to first familiarize yourself with what AI is in order to articulate what you plan to achieve with it.
With a strategic step-by-step approach, and the right partner to guide you, you will be able to go through the process of AI adoption smoothly.
About the writer:
Kasper Halme
SKALER's Operations. Services Lead - Determined to understand complex issues. In a true engineering spirit, likes to solve problems and get things done.